Generative AI is revolutionizing the energy industry with numerous applications that enhance efficiency, sustainability, and operational performance. If you’re interested in learning more about the fundamentals of Generative AI, check out our previous blog where we cover the basics of the technology. In this article, we will explore how Generative AI is currently applied in the energy industry, discuss its future potential, and address some of the challenges it faces.
Generative AI holds immense promise for businesses that can fully leverage data analysis and automation. Its power lies in its ability to process and learn from large volumes of data, generating impactful insights. One of the most effective applications of Generative AI is in energy management.
Energy Efficiency
Traditional energy management in industrial organizations relies on a deep understanding of a facility’s energy consumption and how that consumption can be reduced or shifted with minimal impact on production. This type of data analysis is what generative AI excels at, as optimizing energy efficiency is essentially pattern recognition. One of the most common applications of generative AI in the energy industry today is helping facilities optimize their energy usage by identifying high-energy consuming assets and suggesting load shifts based on grid dynamics.
Load Optimization
Feeding AI with real-time facility data and live grid data reveals the true value of generative AI. Load optimization allows you to consider variables such as energy pricing, production data, and asset-level energy consumption to maximize efficiency. Manual load optimization is time-consuming and less effective because it requires analyzing many changing variables (grid data, consumption, facility requirements, etc.). When a change is needed, the optimization process must be redone. However, with generative AI, continuous monitoring and analysis of data enable swift adjustments to load scheduling.
Edgecom Energy is actively developing this technology and recently won the ABB Startup Challenge 2024 for our AI-driven Load Optimization Engine. As a result, we are now collaborating with ABB to integrate our AI Energy CoPilot into ABB Ability™.
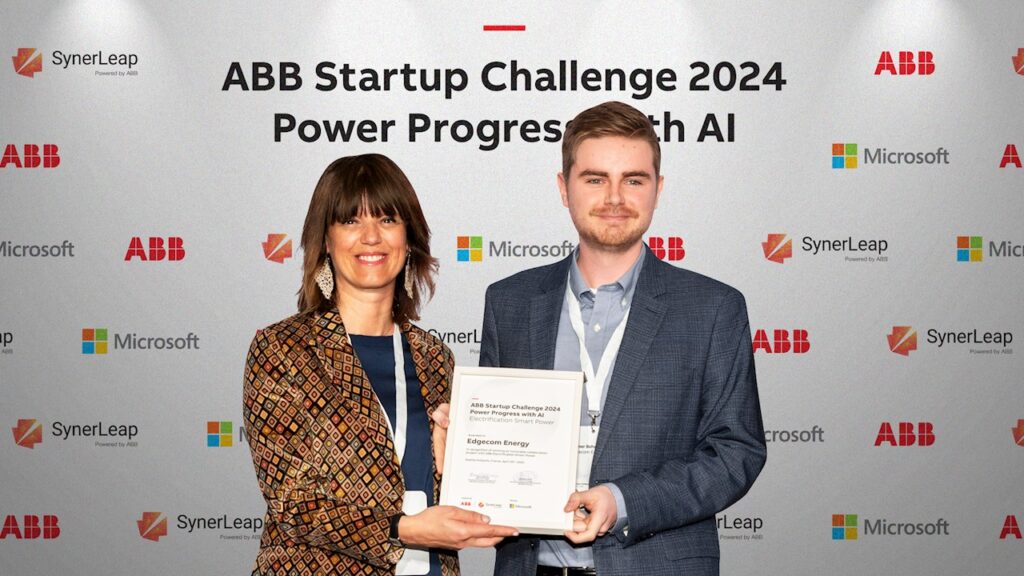
Peak Forecasting
Many peak forecasting solutions including pTrack® utilized machine learning algorithms to forecast peaks long before Generative AI became widespread. Where Gen AI comes in is taking those existing algorithms and transforming them into actionable curtailment and response strategies.
Sustainability and Carbon Reduction
Energy consumption and carbon emissions are closely linked. Processes that enhance energy and operational efficiency also help reduce carbon emissions. Generative AI can help strategically develop sustainability plans based on your facility’s actual data. It also simplifies ESG reporting by making all your energy and emissions data, along with useful insights, instantly available through the AI.
Future Applications of Generative AI
Automation and Control
One of the most exciting prospects for generative AI is its potential to control processes. Currently, most generative AI applications focus on data analysis and insights. The next evolution will enable these applications to control processes based on derived insights. This could include automatic load shifting in production, pre-cooling facilities during non-peak hours, or even more advanced processes such as automatically switching facilities to distributed energy resources based on real-time grid dynamics.
Predictive Maintenance
With historic and real-time data, Generative AI can begin to recognize abnormal patterns in your assets and inform you of maintenance needs. By preemptively identifying faults and failures before they occur, you can reduce downtime, minimize failures, and ultimately lower costs. Combined with automation, detected abnormalities can trigger automatic notifications to maintenance teams or update the maintenance schedule.
Challenges of Generative AI
The Energy Cost of AI
One of the biggest challenges in utilizing generative AI in the energy industry is the energy cost of AI itself. Generative AI consumes a large amount of energy due to its substantial computational requirements. According to Google’s 2024 Environmental Report, global data center electricity consumption is estimated to be 240-340 TWh, which accounts for roughly 1-1.3% of global electricity demand. With AI driving the need for increased data storage and computational power, Microsoft, Google, and Amazon are collectively investing over $250 million USD in data center infrastructure to support the AI boom. Fortunately, sustainability is a key focus of these investments and below represents the sustainability commitment these companies have made
Initiative | Amazon | Microsoft | |
---|---|---|---|
Net-Zero Carbon | Achieve net-zero carbon by 2030. | Achieve net-zero carbon by 2040. | Achieve net-zero carbon by 2030. |
Renewable Energy | Run on 24/7 carbon-free energy (CFE) on every grid by 2030. | Power operations with 100% renewable energy by 2025. | Use 100% renewable energy by 2025. |
Water Stewardship | Replenish more water than consumed by 2030. | Replenish more water than consumed by 2030. | Replenish more water than consumed by 2030. |
GHG Emissions | Reduce 50% of combined Scope 1, 2, and 3 emissions by 2030 (from 2019 base) | Reduce Scope 1 and Scope 2 emissions by 50% by 2030. | Reduce Scope 1, 2, and 3 emissions by 50% by 2030 (from 2020 base) |
Circular Economy | Achieve zero waste to landfill for global data center operations. | Eliminate single-use plastics in packaging by 2025. | Achieve zero waste certification for direct operations by 2030. |
Ongoing research and development aim to improve the efficiency of AI models and combined with more efficient next-generation datacenters, the energy cost of AI will likely decrease in the future.
Hallucinations
Hallucinations in generative AI refer to incorrect or nonsensical answers produced by the model. These occur because the AI generates responses based on patterns learned from its training data, rather than having a true understanding or access to real-time information. The model relies on probabilities and patterns rather than actual comprehension. As AI models are trained on higher-quality data, incorporate feedback mechanisms, and undergo architectural improvements, the frequency of hallucinations is expected to decrease. It’s important for users to fact check generative AIs in its current state and not solely rely on its output.
Data Security
In today’s digital age, data security is a huge concern and generative AI has only highlighted this fact more due to the vast amount of data being used to train AI models. When engaging with AI models, it’s important to be cognizant of providing sensitive information to the AI. Ask yourself these questions:
- Is my data encrypted?
Encryption is a fundamental aspect of data security, ensuring that your data is encoded in a way that makes it inaccessible to unauthorized users. Confirm whether the AI provider uses strong encryption protocols for data in transit (as it moves between your device and their servers) and at rest (when stored on their servers). This helps protect your data from potential breaches and unauthorized access. - How is my data stored and processed?
Understanding the methods used to store and process your data can reveal potential risks. Inquire about how long your data is retained, whether it is stored in secure environments, and the processes in place for data management. Secure storage practices and robust data handling procedures are essential for minimizing the risk of data exposure or misuse. - Do any third-party companies have access to my data?
It’s important to know if your data is shared with or accessible by third-party entities. Some AI services may partner with other companies for analytics, marketing, or other purposes. Ensure that any third parties involved adhere to strict data protection standards and that you are informed about their roles in handling your data.
By address these questions and becoming knowledgeable about the AI models you interact with, you can better safeguard your data and ensure.
You can learn more about how LLMs are transforming energy management through our free webinar, which includes a live demo of our AI Energy CoPilot: